isolated Structure of phospholipid molecules 3d rendering | Image Credit: © Love Employee - stock.adobe.com
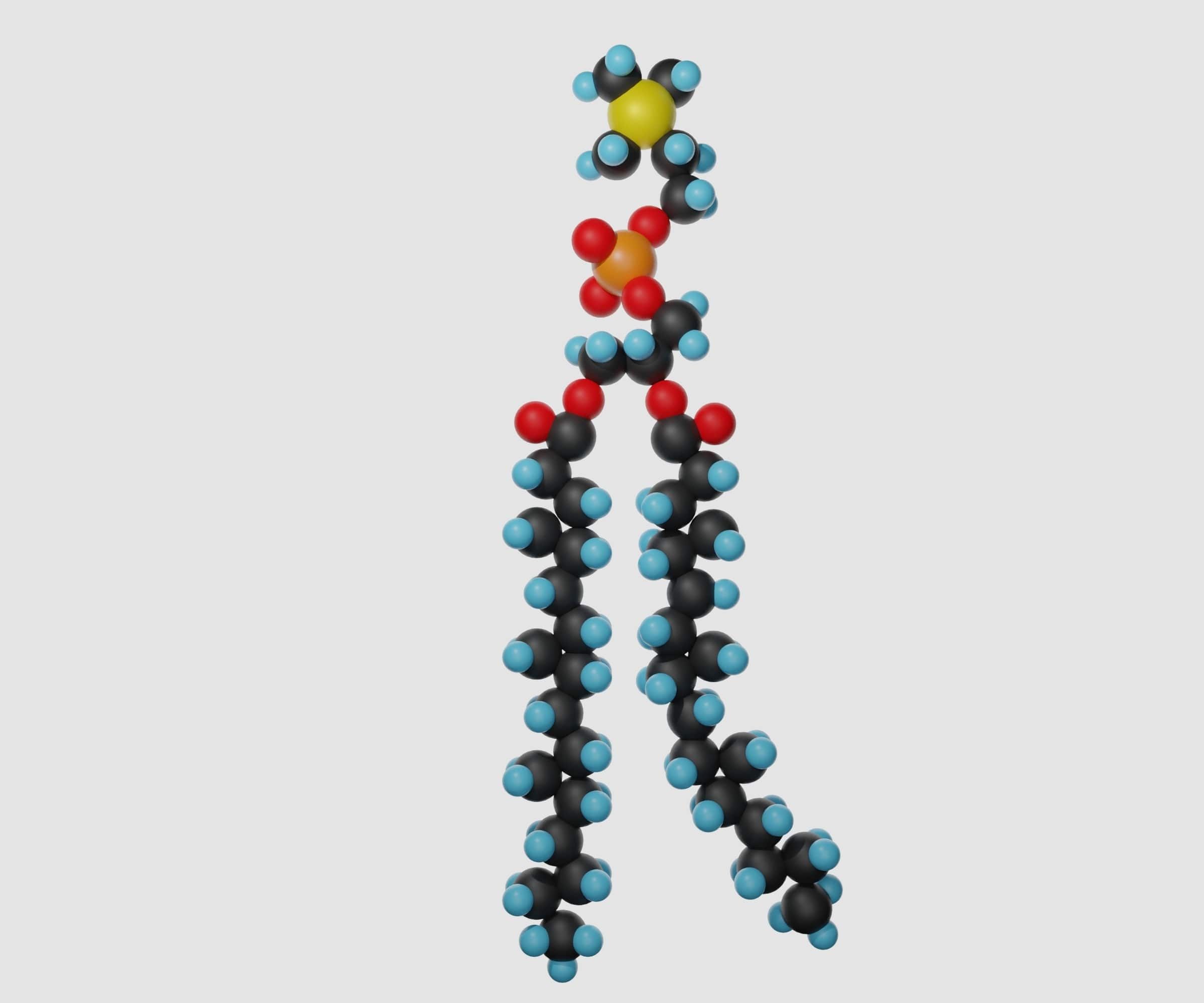
In a recent study out of the University of Gdańsk, immobilized artificial membrane chromatography (IAM) was tested for its ability to evaluate small molecular behavior in the human body.
A recent study conducted by Krzesimir Ciura, an assistant professor from the University of Gdańsk, introduced a new model that advances drug discovery, especially in the discipline of molecular analysis. Ciura’s study, which was published in the Journal of Chromatography A, explores the use of immobilized artificial membrane chromatography (IAM), and how it could be used to assess the molecular behavior in the human body (1).
isolated Structure of phospholipid molecules 3d rendering | Image Credit: © Love Employee - stock.adobe.com
The purpose of the study was to test whether IAM chromatography could be an improved method for measuring lipophilicity. To determine whether IAM, which could serve as a biosimilar alternative, can measure lipophilicity better than existing methods, Ciura integrated the Quantitative Structure-Retention Relationships (QSRR) approach with mechanistic descriptors, employing advanced Chemicalize software (1). Using this software led to the generation of a large, varied data set. Anticipating this result, Ciura used locally weighted least squares kernel regression, a similarity-based machine learning method, to sort through the data (1).
Ciura’s findings led to the conclusion that three factors are wholly responsible and important in determining molecule binding to phospholipids, and these factors are lipophilicity, charge, and maximum projection area (1). Ciura also validated the created model following OECD guidelines, which showed that the model had great predictive capabilities with a high predictive squared correlation coefficient (Q2) of 0.812 and a low root mean square error of prediction (RMSEP) of 6.739 (1).
The team leveraged IAM-HPLC data alongside molecular descriptors, formulating the chromatographic hydrophobicity index with immobilized artificial membrane (CHIIAM) as an endpoint in QSRR modeling. Ciura created a QSRR model that had the ability to analyze 402 molecules, predominantly of pharmaceutical or toxicological relevance (1). Validation of this model involved assessing retention factors of 106 potential drug candidates across five distinct chemical classes. The Chemicalize software enabled the calculation of mechanistic molecular descriptors, facilitating the interpretation of the QSRR models (1).
Predicting drug behavior requires an understanding of the affinity between small xenobiotics and phospholipids (1). With better methods to predict drug behavior, it makes it easier for scientists to discover and develop new drugs that could be beneficial in treating specific illnesses. This new approach offers a new way to expedite the drug discovery process. It offers a nuanced understanding of molecular interactions crucial for developing safer and more effective pharmaceuticals (1).
This article was written with the help of artificial intelligence and has been edited to ensure accuracy and clarity. You can read more about our policy for using AI here.
(1) Ciura, K. Modeling of Small Molecule's Affinity to Phospholipids Using IAM-HPLC and QSRR Approach Enhanced by Similarity-based Machine Algorithms. J. Chromatogr. A. 2024, 1714, 464549. DOI: 10.1016/j.chroma.2023.464549